As AI’s power grows, humans need to be kept in the loop, say Terence Tse, Emily Liao, Danny Goh and Mark Esposito.
There is no doubt that the increasing use of artificial intelligence (AI) can make our lives easier. However, the benefits of AI adoption are accompanied by many new problems. One is that machine learning algorithms typically operate like ‘black boxes’: we can only see the inputs and outputs, not how those inputs are being combined to reach results. The machines are making predictions in ways that are completely unobservable to us.
That throws up numerous issues. Applying black box algorithms in many areas of public life, such as in the justice system, would have deep social and ethical ramifications. Nor is such opaqueness acceptable for businesses. How can managers justify using algorithms without being able to clearly and fully explain how they work? Machine learning technologies are advancing at full speed, but the methods for monitoring and troubleshooting them are lagging behind (see, for instance, Deloitte’s Future of Risk in the Digital Era paper from 2019).
As AI becomes more pervasive and sophisticated, it is being entrusted with business-critical decisions – but companies which ignore the black box problem are setting themselves up for potential issues which could be costly and performance-impacting, resulting in operational inefficiencies, reputational damage or even legal repercussions. They will also find it tricky to determine the causes of errors and make refinements to their AI setup. What organizations need to consider is ‘white box AI’.
Let there be light
At the centre of white box AI is the idea of a system that is understandable, justifiable and auditable by humans. The belief that ‘the more powerful the AI model, the better the resulting business offering’ is common, but erroneous. The true power of AI does not lie in the prowess of models. It comes from having the right business setup and infrastructure that can make the best use of the models’ output. (See ‘The dumb reason your AI project will fail’, Harvard Business Review, June 2020). A simple model that operates in a system where the workings are transparent triumphs over far more sophisticated models that we cannot see into.
To make white box AI a reality, managers must apply the idea of the ‘expert-in-the-loop’ (EITL) – a concept which builds on that of the ‘human-in-the-loop’ (HITL). HITL refers to AI-driven systems in which humans retain full control and their oversight is active and involved (see box above). HITL isn’t required in every instance: for many tasks it will be possible, and make business sense, to use algorithms to fully automate routines with a well-defined end goal. One example is ‘eye-balling’ the details on PDF documents and keying them into a computer.
Yet businesses gain their advantages from well-designed workflows and processes which cannot be easily, if at all, replicated with AI. In these cases, the answer is making humans part of the system. This mitigates most of the downsides related to black box AI, and promotes accountability and explainability – vital for AI-enabled decisions in many industries, including financial services and healthcare.
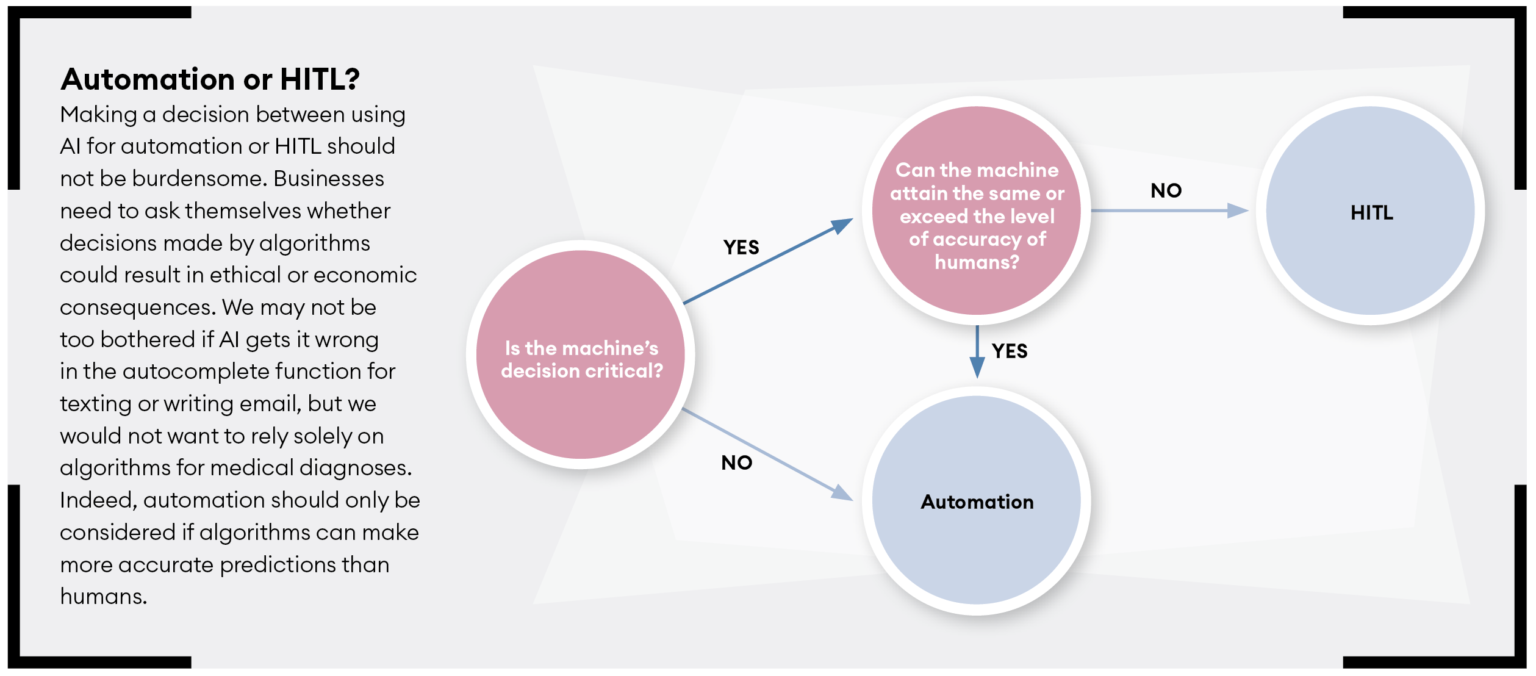
The human-machine relationship
As the name suggests, EITL takes a further step up from HITL. Typically, businesses contain three types of experts: the technical specialists who deal with model design, training, operationalization and deployment; the subject matter experts who own the business problem to be solved; and the end-users of the output, such as customer-facing staff.
What makes EITL different is the interactivity among these experts, allowing their feedback to drive overall system improvements. Information loops which take input from the experts and combine it with real-time system data can be used to fine-tune the model. Our practical experience of AI initiatives has shown that successful deployment is often the product of such improvement loops. For example, the expert compliance team of one of our clients, a global bank, is now supervising the algorithms that we designed and built for them to check customers’ personal documents, instead of manually performing the checks themselves. Working with the technical experts, the team reviews and corrects algorithm outputs, which leads to improved model accuracy and a more effective and efficient sales quality checking operation.
Additional advantages of the EITL structure include mitigation of model bias risk and increasing sustainability and scalability. AI technologies themselves can be difficult to upgrade to meet ever-changing consumer demand. However, with an architecture wherein outputs are validated by humans, corrections can always follow requirements. With these benefits in place, businesses can rest assured that they will be better accountable for their AI-enhanced decision-making.
White box AI is not a breakthrough in and of itself. Rather, it is a proven means to an end: for keeping pace with inevitable technological advancement in the AI space. As more and more algorithms are used in potentially sensitive aspects of our lives, the transparency and accountability of algorithm-driven systems is paramount. By leveraging the expertise of human actors with an EITL setup, companies will be in a much better position to realize the full potential of AI while remaining firmly in control of their technologies.
This article is also featured on Dialogue Review, and Duke Corporate Education, and is written by Danny Goh, Emily Liao, Mark Esposito, and Terence Tse.
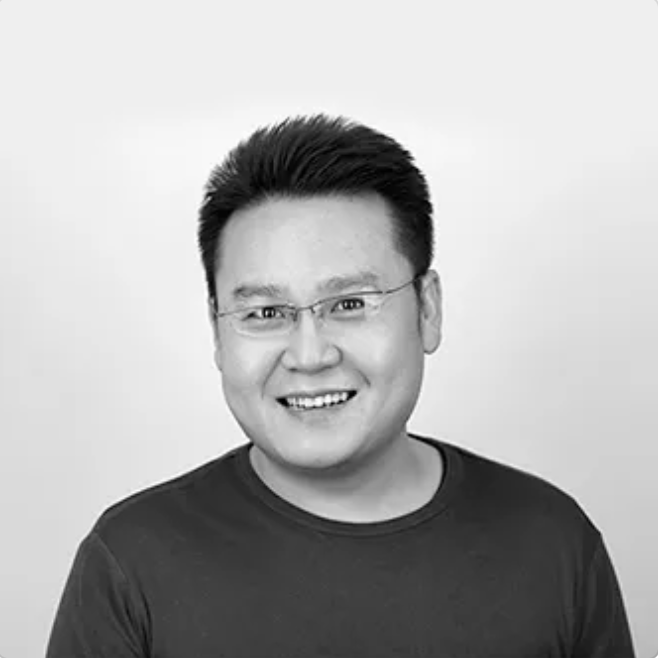
Danny Goh
Serial entrepreneur and an early-stage investor, co-founded and been CEO of Nexus FrontierTech, investing in early-stage start-ups with 20+ portfolios; currently serves as an entrepreneurship expert with the Entrepreneurship Centre at Said Business School, University of Oxford.
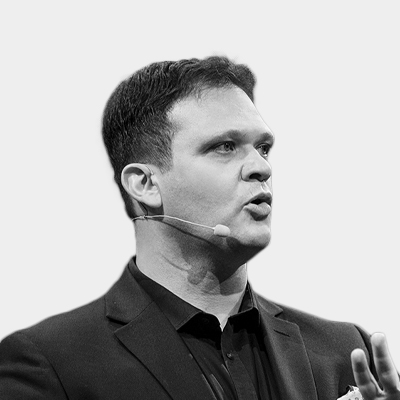
Mark Esposito
Professor of business and economics at Hult International Business School and at Thunderbird Global School of Management at Arizona State University; a faculty member at Harvard University since 2011; a socio-economic strategist researching the Fourth Industrial Revolution and global shifts.
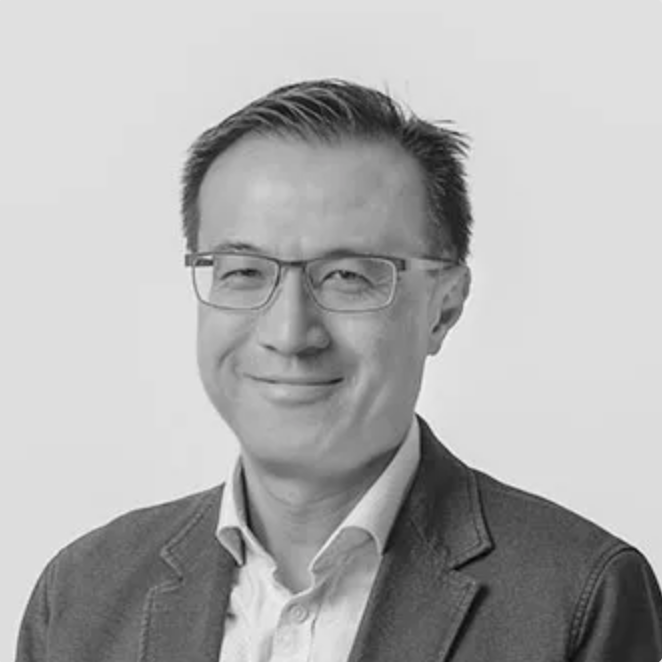
Terence Tse
Professor at ESCP Business School and a co-founder and executive director of Nexus FrontierTech, an AI company. He has worked with more than thirty corporate clients and intergovernmental organisations in advisory and training capacities.
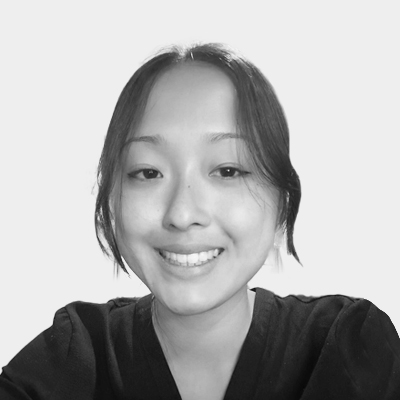