What are the natural extensions of machine learning (ML) and deep learning as well as natural language processing (NLP) and affective computing (AC)?
To many people, what distinguish machines from humans is emotion. True, some existentialists might push the envelope and go so far as to say consciousness (which is a valid argument), but the primary existential reality is emotion. A computer is not a living entity, does not understand empathy and cannot gauge how we feel. It does not and cannot care whether its users are happy, sad, frustrated or simply regretting a heavy lunch.
But the subject of emotions is a pertinent one as it defines most of our decisions. Recent advances in social cognitive neuroscience and behavioural science now prove that rationality is only one of the governing principles in decision making. We are also affected by moods and consult our ‘gut feeling’ about an investment. If we were ruled by pure reason, then why would we engage in charity and philanthropy?
Emotions and language, which are one of the ways in which we convey emotions, thus play an important role in the way investment decisions are executed. Owing to this, considerable progress has been made in recent years in understanding the subtleties of language and emotion. These areas of study are referred to as NLP and AC respectively.
Natural language processing
In our previous research, we have largely focused on the quantitative methods of analysis. However NLP is more qualitative in nature. While quantitative data is easier to compartmentalize in the form of, say, share prices, time-series data analysis, qualitative data is harder to define and statistically model.
For example, when Facebook was about to become a publicly listed company in 2012, how was it that company that was earning $1 billion in revenue was given a market valuation of $90 billion? The reason for this was based on several qualitative factors such as its core ideas, its teams, and its projected potential to earn high revenue, etc. In this view, qualitative data plays an important role even in financial decisions. In fact, structured quantitative data in the form of spreadsheets and relational databases only account for 20% of all available data. The remaining 80% is in the form of social media posts (especially Twitter), images, email, text messages, audio files, Word documents, PDFs and other unstructured forms.
To extract insights from these unstructured sources of data, NLP uses ML and AI to understand unstructured text and provide context to language. This allows us to parse information (summarize, reduce or categorize qualitative data) being received via announcements, rumours, expert forecasts etc. in real-time and hone the decision-making process from the barrage of online information.
In terms of interpreting this qualitative information, NLP can be broken down into the following sub-topics:
- Signal processing: the conversion of spoken words into text.
- Syntactic analysis: what is the structure and grammar of the sentences
- Semantic analysis: what is the meaning of the words. What is the logic of the sentences.
- Pragmatics: what is the context of the sentences. How is it related to the rest of the sentences.
Most NLP is based on the last three. Recent advances in each of these sub-topics has allowed us to use NLP to a deeper understanding of public perception of products, services, brands and companies.
Sentiment thus plays a very important role in decision making and the ability of a machine to convert human language into machine readable code and convert it into actionable insights is the capability offered by NLP.
Affective computing
The topic of sentiment brings us to AC. While NLP is capable of reading or converting words into a stream of logic that can be used as an input to a computation devise, there are subtle nuances that humans use to communicate. Sentiments can be deciphered if they are written based on the structure of the words, the meaning of the sentence and even the speed at which it is written (do you type faster or slower when you are angry?)
But language is only one way of exhibiting emotions. We also tend to use our bodies. A head shake can mean agree, disagree, confused, or simply a stiff neck. While it is relatively easy for us to decipher the meaning of these gestures (depending on which cultural context we are immersed in), automating this task to a computer is a true challenge for scientists trying to bridge the barrier between machine-human interfaces. This has led to the development of AC.
AC is the study and development of systems and devices that can recognize, interpret, process and simulate human affects. It is an interdisciplinary field spanning computer science, psychology, and cognitive science that attempts to sense the emotional state of a user using sensors and deep learning.
Much like in the way a deep learning algorithm is fed thousands and thousands of images capable of identifying variations of how the number can be written, AC uses a similar method to learn how a face is smiling – instead of numbers, feed the computer with tons of data of what a smile looks like for men, women and children of any nationality and color.
Affective computing is the next step in the analysis of sentiments and emotions. At a recent conference at MIT experts forecasted that it will affect all areas of industry from advertising and market research to consumer products. As facial expressions and spoken words can now be observed and analyzed in real time, we are now entering an era in which physical responses to stimuli can be identified and emotions can transformed into measurable results providing us with guidelines to what actions need to be taken.
Business implications
Chatbots are already adopting AC. They are programs that mimic conversation with people using artificial intelligence (AI). With recent advances in AI, chatbots have become accurate conversationalists, especially when focused on a specific domain. As increasing number of consumers prefer to text, chatbots are increasingly becoming the de facto mode of communicating with a website. Rather than have a client helpline or a customer service helpline, chatbots offer a cheaper and easier mode of communication.
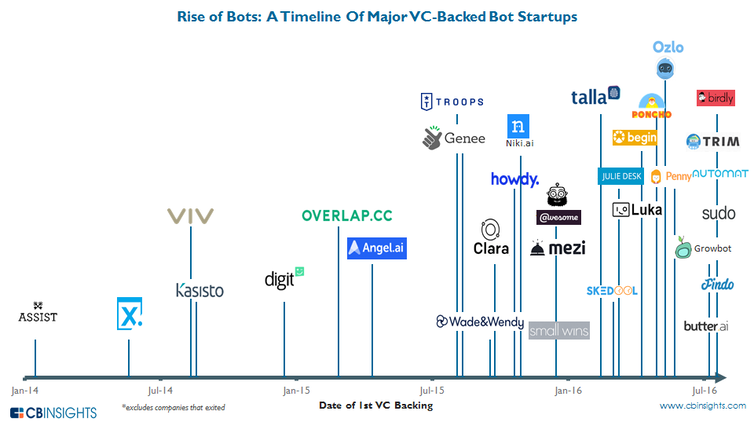
The uses of AC in the world of business are yet to be fully explored. One possibility is that if you are prone to stress or work in a high stress environment that is detrimental to your health, then sensors that can measure your emotional state and gauge what you are feeling. This information can be used as input data to modify your insurance plan as insurers can now know what the stress levels and potential health risks of their insured are.
This article is also featured on The Conversation, and is written by Mark Esposito, and Terence Tse.
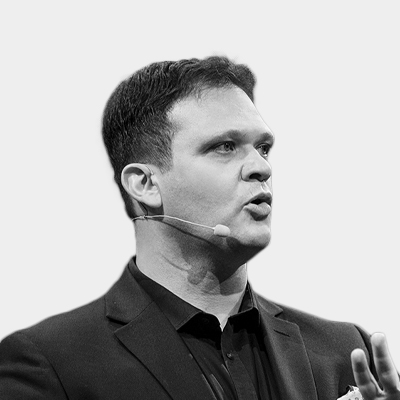
Mark Esposito
Professor of business and economics at Hult International Business School and at Thunderbird Global School of Management at Arizona State University; a faculty member at Harvard University since 2011; a socio-economic strategist researching the Fourth Industrial Revolution and global shifts.
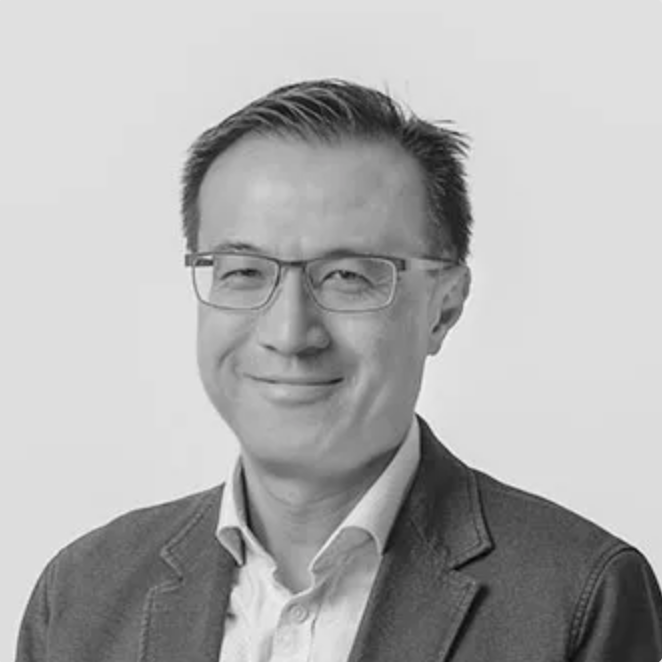
Terence Tse
Professor at ESCP Business School and a co-founder and executive director of Nexus FrontierTech, an AI company. He has worked with more than thirty corporate clients and intergovernmental organisations in advisory and training capacities.