Our lives are ruled by data. Not just because it informs companies of what we want, but because it helps us to remember and differentiate what we want, what we need, and what we can ignore. All these decisions give way to patterns, and patterns, when aggregated, give us a picture of ourselves. A world where such patterns follow us, or even are sent ahead of us — to restaurants to let them know if we have allergies, to retail stores to let them know our preferred clothing size —is now so feasible that labeling it science fiction would expose a lack of awareness more than a lack of imagination.
The benefits of AI are making many of our choices easier and more convenient, and in so doing, tightening competition in the space of customer choice. As this evolution happens, the question is less to what extent AI is framing our choices, but rather, how it is shaping them. In such a world, we need to understand when our behavior is being shaped, and by whom.
Clearly, most of us are quite comfortable living in a world where our choices are shaped by AI. Indeed, we already live in such a world: from search engines to smooth traffic flow, many of our daily conveniences are built on the speed provided by the backend. The question we need to ask ourselves when considering AI, and its governance, is whether we are comfortable living in a world where we do not know if, and how, we are being influenced.
Behavioral Bias or Behavioral Cues?
AI can do for our understanding of behavior what the microscope did for biology.
We have already reached the point where software can discover tendencies in our behavioral patterns we might not consider, identifying traits that our friends and family would not know. The infamous, but apocryphal, story of the father who discovered his daughter was pregnant when Target began sending advertisements for baby suppliers (after detecting a shift in her spending) gives us a sneak peek.[i]
Our lives are already ruled by probabilistic assumptions, intended to drive behavior. Now we need to ask, and answer honestly, how much of your life are you willing to have shaped by algorithms you do not understand? More importantly, who should be tasked with monitoring these algorithms to flag when they have made a bad decision, or an intentionally manipulative one?
As more companies use AI, and the complexity of its insights continues to grow, we will be facing a gap above the right to an understanding, or the right to be informed – we will be facing a gap concerning when, and if, a violation has occurred at all.
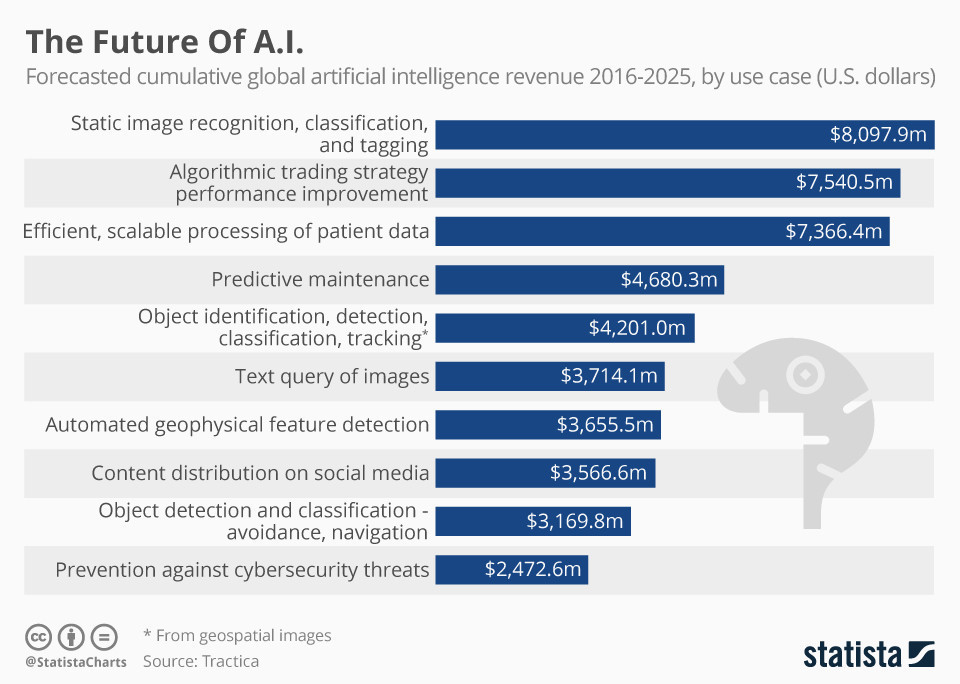
As our digital presence grows, and this presence is being pulled by public directions for the future of e-governance and private for how we engage with our interests – meaningful governance will have to include an essential first step, the right to know how our data is being used, who has it, and when are they using it.
Another example of how behavior and technology are interfacing at a faster than ever pace is through the observation of what Chatbots have been shown to provide us: the potential for emotional associations, which might be used for manipulative purposes.[ii] As developments in natural language processing grow to combine with advanced robotics, the potential of building that bond from touch, warmth, comfort, also grows – particularly in a world where we experience the largest endemic of loneliness, driving the UK to literally appoint a minister for loneliness.
As machine to machine data grows in the internet of things, companies with preferential access will have more and more insight into more and more minute aspects of behavioral patterns we ourselves might not understand — and with that comes a powerful ability to nudge behavior. Good data is not just about volume, it is about veracity — as IoT grows, we are handing firms everything they need to know about us on a silver platter.
We can argue still that the issue is not the volume, the issue is the asymmetry of analytic competency in managing that volume — meaning asymmetries in capturing value. In turn, this means some companies not only understand you, but can predict your behaviour to the point of knowing how to influence a particular choice most effectively. In the age of big data, the best business is the insight business.
Accountability: who is looking after us?
The first question concerning building accountability is how to keep humans in the decision loop of processes made more autonomous through AI. The next stage needs to preserve accountability in the right to understanding — to know why an algorithm made one decision instead of another.
New proposals are already emerging on how to do this — for example, when specific AI projects are proprietary aspects of a firm’s competitiveness, we might be able to use counterfactual systems to assess all possible choices faced by an AI.[iii] But systems that map decisions without breaking the black box will not be able to provide the rationale by which that algorithm made one decision instead of another.
Yet the problem still goes deeper. The problem with transparency models is the assumption that we will even know what to look for — that we will know when there needs to be a choice in opting out of a company’s use of data. In the near future, we may not be able to understand by ourselves when an AI is influencing us.
This leads us to a foundational issue: to govern AI, we may need to use AI.
We will need AI not just to understand when we are being influenced in overt ways, but to understand the new emerging ways in which companies can leverage the micro-understanding of our behavior. The capacity for existing legal frameworks, existing political institutions, and existing standards of accountability to understand, predict, and catch the use of AI for manipulative purposes is sorely lacking.
Algorithmic collusion is already a problem — with pricing cartels giving way to pop-up pricing issues that can disappear, without prior agreement, thus avoiding the initial claims.[iv] We can imagine a world where collusion is organized not by the market, but by tracking the behavior of distinct groups of individuals to organize micro-pricing changes.
Naturally, questions emerge: who will govern the AI that we use to watch AI? How will we know that collusion is not emerging between the watchers and the watched? What kind of transparency system will we need for a governing AI to minimize the transparency demands for corporate AI?
The future of AI governance will be decided in the margins — what we need to pay attention to is less the shifting structure of collusion and manipulation, but the conduct, and the ability for competent AI to find the minimal number of points of influence to shape decision making.
We need to have a conversation to make our assumptions and beliefs about price fixing, about collusion, about manipulation, painfully clear. In an age of AI, we cannot afford to be vague.
This article is also featured on World Economic Forum, and is written by Danny Goh, Mark Esposito, and Terence Tse.
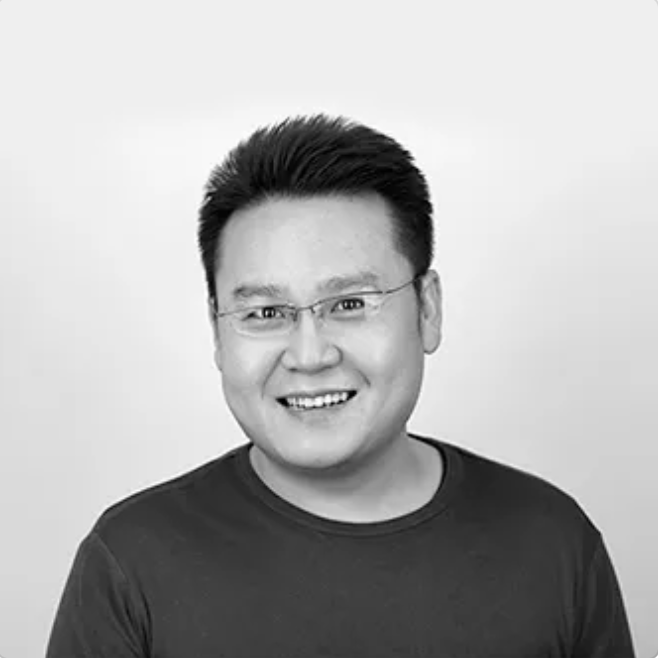
Danny Goh
Serial entrepreneur and an early-stage investor, co-founded and been CEO of Nexus FrontierTech, investing in early-stage start-ups with 20+ portfolios; currently serves as an entrepreneurship expert with the Entrepreneurship Centre at Said Business School, University of Oxford.
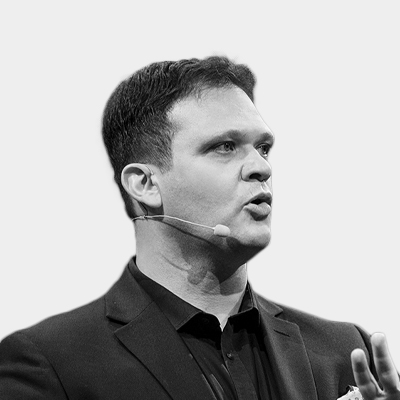
Mark Esposito
Professor of business and economics at Hult International Business School and at Thunderbird Global School of Management at Arizona State University; a faculty member at Harvard University since 2011; a socio-economic strategist researching the Fourth Industrial Revolution and global shifts.
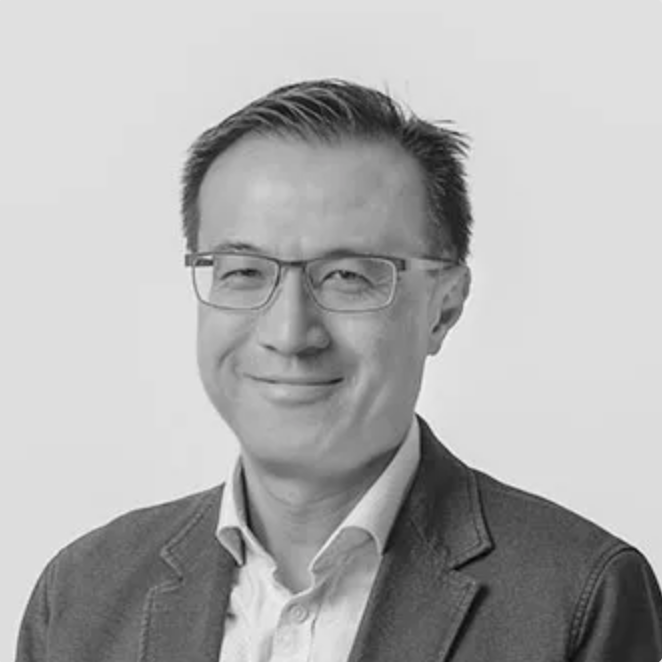
Terence Tse
Professor at ESCP Business School and a co-founder and executive director of Nexus FrontierTech, an AI company. He has worked with more than thirty corporate clients and intergovernmental organisations in advisory and training capacities.